AI and Machine Learning in Automation
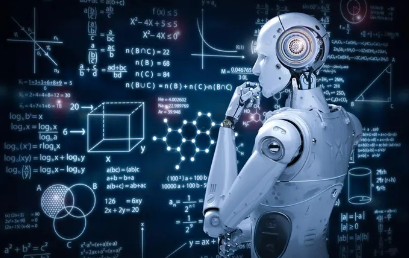
In the realm of automation, AI and Machine Learning act as the unseen architects shaping the future of industries. Imagine a world where processes adapt, decisions are optimized, and maintenance is predictive. The synergy between AI and Machine Learning not only revolutionizes traditional workflows but also opens doors to new possibilities. As you explore the intricate dance of algorithms and data in automation, you’ll uncover the transformative power that lies within this dynamic duo, propelling businesses towards unprecedented efficiencies and innovation.
Evolution of Automation Technologies
Over the past few decades, automation technologies have undergone a rapid evolution driven by advancements in computing power and software capabilities. From a historical perspective, automation initially focused on repetitive tasks in manufacturing industries. However, with technological advancements, automation has expanded its reach across various sectors such as healthcare, finance, and transportation.
In the industry applications of automation, we’ve witnessed a significant shift towards increased efficiency, higher productivity, and improved quality control. Robots and automated systems are now commonplace in factories, warehouses, and even customer service centers. The integration of automation technologies hasn’t only streamlined processes but also reduced human error and enhanced safety standards.
Looking towards the future implications of this evolution, we can expect even more sophisticated automation systems powered by AI and machine learning. These technologies will enable predictive maintenance, autonomous decision-making, and adaptive learning capabilities, revolutionizing how industries operate. The continued development of automation holds the promise of increased competitiveness, cost savings, and innovation across global markets.
Role of AI in Process Optimization
AI plays a crucial role in optimizing processes by leveraging data-driven insights and automation techniques. When it comes to resource allocation, AI excels in determining the most efficient distribution of resources within an organization. By analyzing historical data and real-time information, AI can predict demand fluctuations and allocate resources accordingly, leading to cost savings and improved operational efficiency.
Machine learning is particularly impactful in supply chain optimization. Through advanced algorithms, AI can analyze vast amounts of data to identify patterns and optimize supply chain processes. By predicting demand, streamlining inventory management, and enhancing logistics operations, AI can help organizations reduce lead times, minimize stockouts, and improve overall customer satisfaction.
Machine Learning for Predictive Maintenance
Utilizing machine learning algorithms enables organizations to predict equipment failures and schedule maintenance proactively. Predictive maintenance offers significant benefits such as reduced downtime, lower maintenance costs, and increased operational efficiency.
By analyzing historical data and identifying patterns, machine learning algorithms can forecast when a machine is likely to fail, allowing for timely intervention before a breakdown occurs.
However, predictive maintenance also has its limitations. It requires vast amounts of high-quality data for accurate predictions, and the algorithms may not always account for unforeseen circumstances or anomalies in the data. Careful consideration must be given to the selection of machine learning algorithms to ensure they’re appropriate for the specific maintenance needs of the equipment being monitored.
Factors such as the type of equipment, data availability, and the desired level of accuracy must be taken into account when choosing the most suitable algorithm for predictive maintenance tasks. Proper algorithm selection is crucial for maximizing the effectiveness of predictive maintenance strategies.
Enhanced Decision-Making With AI
AI empowers precision in decision-making by analyzing vast datasets and identifying intricate patterns.
Machine learning enhances operational efficiency by automating processes and adapting to changing conditions.
Together, AI and ML offer a dynamic duo that can revolutionize how organizations make strategic choices and streamline operations in the realm of automation.
Leveraging these technologies equips businesses with the tools needed to optimize performance and drive continuous improvement.
AI for Precision
With the integration of advanced algorithms and data analytics, precision in decision-making reaches new heights when employing machine learning technology.
AI for precision agriculture optimizes processes by analyzing data on crop health, soil conditions, weather patterns, and more, enabling farmers to make data-driven decisions for improved yields and resource management.
Machine learning in healthcare diagnostics enhances accuracy by analyzing vast amounts of medical data to assist in disease detection, treatment planning, and patient care.
In precision agriculture, AI algorithms can predict crop diseases, optimize irrigation schedules, and recommend suitable fertilizers based on real-time data, ultimately increasing efficiency and reducing waste.
Similarly, in healthcare, machine learning algorithms can analyze medical images, patient records, and genetic information to provide personalized treatment plans, leading to better outcomes and improved patient care.
Leveraging AI for precision empowers industries to make informed decisions with a high level of accuracy and efficiency.
ML for Efficiency
Enhancing decision-making through the utilization of machine learning (ML) technology can significantly increase operational efficiency across various industries. By leveraging ML applications and AI algorithms, organizations can implement optimization techniques that streamline processes and enhance overall performance.
ML plays a crucial role in driving efficiency improvements by analyzing vast amounts of data to generate insights that aid in making informed decisions. Through the use of advanced algorithms, ML models can identify patterns, trends, and anomalies within datasets, enabling businesses to optimize workflows and resource allocation effectively.
Moreover, ML for efficiency goes beyond traditional methods by continuously learning from new data inputs, adapting to changing circumstances, and providing real-time recommendations. This dynamic approach to decision-making empowers businesses to respond swiftly to market fluctuations, customer demands, and operational challenges.
Challenges in Implementing AI Automation
You’re facing challenges in implementing AI automation, including data quality issues that can impact the accuracy and reliability of your automated processes.
Furthermore, the skill gap challenges may require upskilling your workforce to effectively leverage AI technologies.
Integration complexity obstacles might arise when trying to seamlessly incorporate AI systems into your existing infrastructure.
Data Quality Issues
Implementing AI automation poses significant challenges due to the critical nature of data quality issues. Data accuracy and completeness are fundamental aspects essential for successful AI automation. Inaccurate or incomplete data can lead to flawed predictions and decisions.
Consistency in data is equally crucial; variations in data formatting or structure can significantly impact the performance of AI algorithms. Ensuring that data is consistent across different sources and time periods is a key challenge in implementing AI automation effectively.
Moreover, data relevancy plays a vital role in the quality of the insights derived from AI systems. Irrelevant or outdated data can mislead the automation process, resulting in erroneous outcomes. It’s imperative to continuously evaluate and update the data used by AI systems to maintain relevancy and reliability.
Addressing these data quality issues requires a meticulous approach, including robust data governance frameworks, data validation processes, and regular data quality assessments. By prioritizing data accuracy, completeness, consistency, and relevancy, organizations can enhance the effectiveness of AI automation initiatives.
Skill Gap Challenges
Skill gap challenges present a significant hurdle in the successful deployment of AI automation within organizations. As new technologies rapidly evolve, the demand for skilled professionals who can effectively implement and manage AI automation systems continues to grow.
One of the key solutions to bridge this gap is the development of comprehensive training programs tailored to equip employees with the necessary skills to work with AI technologies. Training programs play a crucial role in addressing skill gaps by providing employees with the knowledge and expertise needed to leverage AI tools effectively.
These programs should cover a range of topics, including data analysis, machine learning algorithms, and programming languages specific to AI applications. By investing in robust training initiatives, organizations can cultivate a workforce that’s adept at harnessing the power of AI automation.
To overcome skill gap challenges, companies must prioritize continuous learning and upskilling initiatives to keep pace with the advancements in AI technology. By fostering a culture of learning and providing access to relevant training programs, organizations can position themselves to successfully integrate AI automation into their operations.
Integration Complexity Obstacles
Addressing integration complexity obstacles in AI automation requires a meticulous understanding of system interdependencies and data flow dynamics.
Integration compatibility challenges often arise due to the diverse technologies and platforms involved in automation processes. System interoperability issues can hinder the seamless communication between different software components, leading to data synchronization problems that impede the effective functioning of AI systems.
Scalability barriers further complicate the integration landscape, making it essential to design automation solutions that can adapt and grow with evolving business needs.
Resolving these obstacles demands a structured approach that involves thorough testing, continuous monitoring, and agile development practices to ensure that the various system elements work harmoniously together.
Impact on Labor Market Dynamics
The integration of AI and machine learning technologies in automation processes has significantly altered the dynamics of the labor market. Job displacement is a key issue arising from automation, impacting various sectors and roles. As certain tasks become automated, there’s a need for retraining programs to equip workers with skills relevant to the evolving job landscape.
This shift in job requirements contributes to job polarization, where high-skilled workers benefit from the adoption of AI and machine learning, while low-skilled workers face challenges in finding suitable employment.
Furthermore, wage inequality is exacerbated by automation, as jobs that can be easily automated tend to offer lower wages, widening the income gap between different segments of the workforce. The labor market dynamics are evolving rapidly due to the integration of AI and machine learning, necessitating proactive measures to address job displacement through effective retraining initiatives and to mitigate the effects of wage inequality and job polarization.
AI Ethics and Bias Considerations
Consider the ethical implications and bias considerations inherent in the deployment of AI and machine learning technologies in various applications.
Ethical AI considerations are crucial in ensuring that these technologies are developed and utilized responsibly. Fairness assessment plays a vital role in identifying and addressing biases that may be present in AI algorithms, particularly those that could lead to discriminatory outcomes. It’s essential to implement bias mitigation strategies to minimize the impact of biases on decision-making processes.
Diversity implications must also be taken into account to ensure that AI systems are inclusive and considerate of various demographic groups. By evaluating the representation of different groups in datasets and testing for disparate impacts, organizations can work towards creating more equitable AI solutions. Striving for diversity in data collection and model development can help mitigate the perpetuation of existing biases.
Ethical AI considerations, fairness assessment, bias mitigation strategies, and diversity implications are all integral aspects of fostering responsible and unbiased AI technologies.
Future Trends in Automation Technologies
You’ll explore the upcoming innovations in automation technologies, focusing on AI-driven enhancements that are reshaping processes across industries.
Understanding the impact of Machine Learning (ML) on automation will be crucial as businesses adapt to the evolving landscape of intelligent systems.
These trends are poised to revolutionize the way tasks are automated and optimized for efficiency and accuracy.
Emerging Automation Innovations
As automation technologies continue to advance rapidly, significant trends are emerging in the realm of automation innovations. Robotics integration and AI applications are shaping the landscape of smart factories, leading to remarkable automation advancements.
In the current automation landscape, robotics integration plays a pivotal role in enhancing efficiency and productivity. Collaborative robots, known as cobots, are becoming increasingly prevalent in smart factories. These robots work alongside human operators, leveraging AI applications to perform tasks with precision and speed.
AI applications are revolutionizing automation by enabling machines to learn from data, adapt to new situations, and make decisions without human intervention. In smart factories, AI-powered systems can optimize production schedules, predict maintenance needs, and identify quality issues in real-time.
The convergence of robotics integration and AI applications is driving the evolution of automation technologies towards more autonomous and intelligent systems.
Future trends in automation will likely continue to focus on leveraging these innovations to create flexible, efficient, and responsive manufacturing environments.
AI-Driven Process Enhancements
AI-driven process enhancements are at the forefront of future trends in automation technologies, revolutionizing industries with increased efficiency and adaptability. Cognitive computing plays a pivotal role in this transformation by enabling systems to mimic human thought processes. Through cognitive computing, automation systems can analyze vast amounts of data, learn from patterns, and make decisions based on complex scenarios, enhancing the overall efficiency of processes.
Robotic process automation (RPA) is another key component driving process enhancements. RPA involves the use of software robots to automate repetitive tasks traditionally performed by humans. By implementing RPA, organizations can streamline workflows, reduce errors, and free up employees to focus on more strategic initiatives. These robots can perform tasks with speed and accuracy, leading to significant cost savings and improved operational outcomes.
Incorporating cognitive computing and RPA into automation processes allows businesses to achieve higher levels of productivity, agility, and innovation. As industries continue to embrace AI-driven enhancements, the potential for transforming traditional workflows into intelligent, adaptable systems becomes increasingly promising.
Impact of ML
Machine learning (ML) is poised to have a significant impact on the future trends in automation technologies, revolutionizing how systems learn and adapt to complex data patterns. As ML algorithms become more sophisticated, they raise ethical dilemmas concerning data privacy and security.
Bias in ML models is another critical issue that can perpetuate societal inequalities if not addressed proactively.
The social implications of widespread ML adoption are vast, influencing job markets and skill requirements. While automation can streamline processes and increase efficiency, it may also lead to workforce displacement and the need for upskilling.
Economically, ML’s impact is twofold – it can drive innovation, creating new markets and opportunities, but it can also disrupt traditional industries, potentially widening economic disparities.
Navigating these challenges requires a thoughtful approach that balances technological advancement with ethical considerations.
Integration of Iot With AI Automation
The seamless integration of IoT devices with AI automation systems enhances operational efficiency and delivers real-time insights for informed decision-making. IoT integration with AI synergy allows smart devices to communicate and collaborate intelligently within automated processes. By connecting IoT sensors, actuators, and other devices to AI algorithms, businesses can streamline operations, optimize resource utilization, and proactively address issues.
Through this integration, AI automation can analyze vast amounts of real-time data generated by IoT devices, enabling predictive maintenance, personalized customer experiences, and adaptive production systems. AI algorithms can learn from IoT data patterns, detect anomalies, and trigger automated responses to improve overall system performance.
Furthermore, the combination of IoT and AI empowers organizations to create more responsive and agile environments, where data-driven insights drive strategic decision-making.
As technology continues to advance, the integration of IoT with AI automation will play a pivotal role in shaping the future of industries across the globe.
Business Opportunities in AI Automation
Exploring the vast array of business opportunities presented by automation powered by artificial intelligence (AI) reveals a landscape ripe with potential for growth and efficiency gains. Investment opportunities in AI automation are abundant, with companies looking to capitalize on the technology’s ability to streamline processes, reduce costs, and enhance decision-making.
By investing in AI-powered automation, businesses can gain a competitive advantage by improving productivity, enhancing customer experiences, and unlocking new revenue streams. Leveraging AI algorithms for predictive analytics can provide insights that drive strategic business decisions and optimize operations.
Additionally, automation can help businesses scale their operations efficiently, adapt to changing market demands, and stay ahead of the competition. Embracing AI automation not only opens doors to new possibilities but also allows companies to stay agile in a rapidly evolving business landscape, making it a cornerstone for sustainable growth and success.
Conclusion
In conclusion, the integration of AI and Machine Learning in automation technologies is revolutionizing industries by enhancing efficiency and driving innovation.
According to a recent report by McKinsey, companies that fully embrace AI and automation technology see a 25% increase in productivity and a 50% reduction in errors.
Embracing these advancements will lead to smarter, more adaptive systems that streamline processes, improve productivity, and unlock new business opportunities.